Geospatial modeling is a critical tool in understanding the complex relationships between various elements in our environment. It encompasses the techniques and processes involved in analyzing spatial data to create detailed representations and simulations of geographic phenomena. The importance of geospatial modeling spans multiple fields, including urban planning, environmental science, transportation, public health, and many others. As we navigate a rapidly changing world, the need for effective and efficient decision-making is more pressing than ever, making geospatial modeling an invaluable asset.
Historical Background
Early Developments in Geospatial Technologies
The origins of geospatial technologies can be traced back several centuries, with some of the earliest maps created by ancient civilizations for navigation and territorial delineation. The advent of cartography marked a significant step forward, allowing for more precise representations of geographic areas.
Evolution of Geospatial Modeling Tools and Techniques
Throughout the 20th century, advancements in computing power propelled the development of more sophisticated geospatial modeling tools. The introduction of Geographic Information Systems (GIS) in the 1960s transformed how spatial data was collected, processed, and analyzed. The evolution from static maps to dynamic, interactive models has fundamentally changed the landscape of research and planning.
Key Milestones in the Field
Several milestones have shaped the field, including the launch of remote sensing satellites in the 1970s, which provided invaluable data for environmental monitoring and resource management. The 1990s witnessed the proliferation of commercial GIS software, making these powerful tools accessible to a broader audience. Today, the integration of Artificial Intelligence (AI) and machine learning with geospatial modeling is paving the way for even more innovative applications.
Key Concepts in Geospatial Modeling
Spatial Data
Spatial data refers to data that represents the position and shape of objects in a defined space. It can be categorized into two main types: vector and raster.
– **Vector data** includes points, lines, and polygons, representing discrete features such as roads, rivers, and administrative boundaries.
– **Raster data**, on the other hand, comprises grid cells and is used to represent continuous phenomena, like elevation and temperature.
Sources of Spatial Data
Spatial data is derived from various sources, including:
– Satellite imagery offers a bird’s-eye view of the earth, capturing data over vast areas.
– GPS data provides precise location information for tracking and navigation.
– Surveys collect firsthand geographic information through fieldwork and community input.
Geographic Information Systems (GIS)
Geographic Information Systems (GIS) are powerful frameworks for managing and analyzing spatial data. They consist of four primary components:
– **Hardware**: This includes computers and servers that process and store geospatial data.
– **Software**: Programs like ArcGIS, QGIS, and GRASS GIS create visualizations and analyses.
– **Data**: A vast repository of spatial and non-spatial data utilized in modeling.
– **People**: Skilled professionals who interpret data and generate insights.
Spatial Analysis Techniques
Spatial analysis is central to geospatial modeling and employs various techniques, including:
– **Buffering**: Determining areas within a specified distance from a feature, useful for assessing impact zones.
– **Overlay Analysis**: Combines different spatial datasets to identify interactions, such as land use changes.
– **Spatial Interpolation**: Estimating values of a variable at unmeasured locations based on known data points, essential for environmental modeling.
Importance of Geospatial Modeling
Applications Across Different Sectors
The applications of geospatial modeling are extensive, including:
– **Urban and Regional Planning**: Identifying growth patterns and optimizing land use.
– **Environmental Management and Resource Conservation**: Monitoring natural resources and assessing environmental impacts.
– **Disaster Response and Recovery**: Analyzing risk areas to improve emergency preparedness strategies.
– **Transportation and Infrastructure Development**: Planning transportation networks and infrastructure projects efficiently.
– **Public Health and Epidemiology**: Tracking disease spread and planning health interventions.
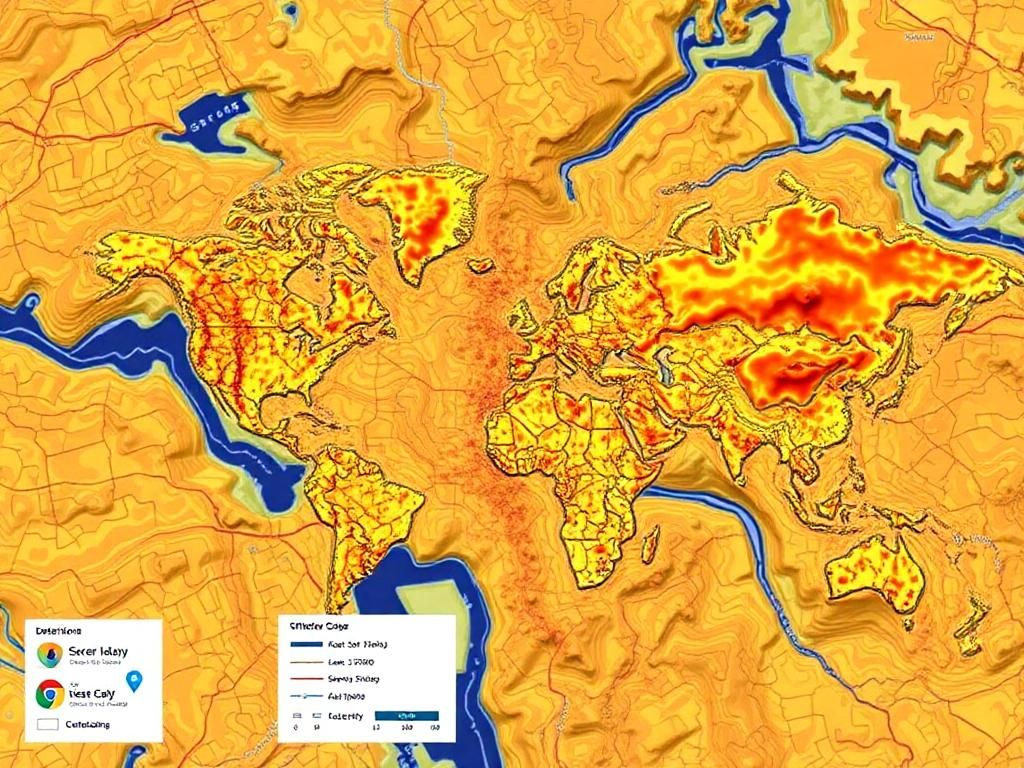
Benefits of Using Geospatial Modeling
Incorporating geospatial modeling into decision-making processes offers numerous benefits, such as:
– Enhanced decision-making capabilities: Data-driven insights enable more informed choices.
– Improved resource management: Efficient allocation of resources reduces waste and maximizes effectiveness.
– Predictive analytics for future planning: Anticipating future trends aids in proactive strategy development.
Tools and Technologies in Geospatial Modeling
Software Solutions
Several software platforms facilitate effective geospatial modeling. Popular ones include:
| Software | Primary Features | Best Use Cases |
| — | — | — |
| ArcGIS | Comprehensive analytics, data visualization | Professional, large-scale projects |
| QGIS | Open-source, user-friendly interface | Community mapping, small businesses |
| GRASS GIS | Advanced modeling, scripting capabilities | Scientific research, complex analyses |
Emerging Technologies
Innovations like AI and machine learning are revolutionizing geospatial modeling by enabling:
– Automated data processing and analysis.
– Enhanced predictive modeling capabilities.
Additionally, remote sensing technologies, including drones and satellites, provide real-time data to improve modeling accuracy. The integration of big data into analytics allows for analyzing larger datasets, leading to richer insights.
Challenges in Geospatial Modeling
Data Quality and Availability
Data quality is paramount in geospatial modeling. Issues such as inaccuracies, outdated information, and gaps can significantly impact modeling outcomes. Ensuring data completeness and reliability is critical for effective analyses.
Technical Challenges
The complexity of modeling processes can pose significant challenges. High computational requirements necessitate robust hardware and software capabilities, potentially limiting access for smaller organizations or researchers.
Ethical Considerations
Geoethical concerns arise relating to privacy, data security, and the potential misuse of spatial data. Furthermore, biases in data collection and interpretation can lead to flawed decisions, underscoring the necessity for ethical practices in geospatial modeling.
Future Trends in Geospatial Modeling
Innovations and Developments
Expect advancements in geospatial analytics and visualization techniques, improving how data is displayed and interpreted. Furthermore, increased integration of the Internet of Things (IoT) will facilitate real-time data analysis, enhancing the relevance and accuracy of models.
Industry Outlook
The growing emphasis on climate change and sustainability will position geospatial modeling as a critical tool in studying environmental impacts and developing solutions. Additionally, as cities evolve into smart cities, the role of geospatial modeling will be pivotal in urban development and infrastructure management.
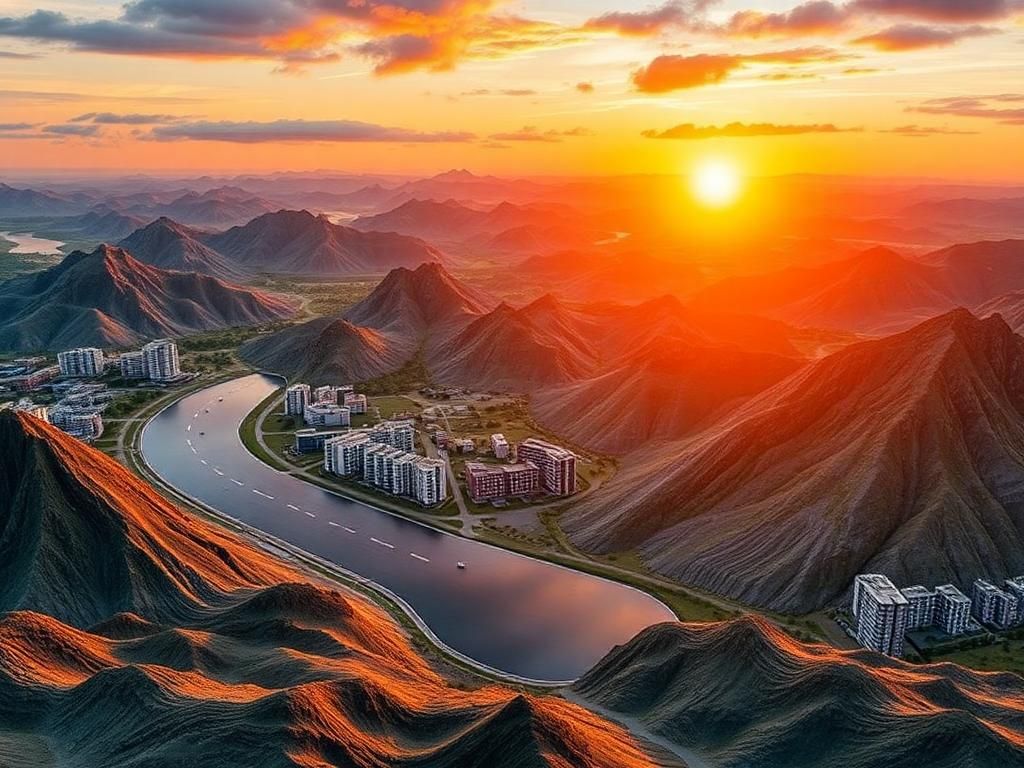
Conclusion
Summary of Key Points
Geospatial modeling represents a transformative approach to understanding and solving complex spatial problems. By integrating advanced technologies and analytical methods, it empowers various sectors to make data-driven decisions that promote sustainability and efficiency.
Call to Action
Professionals across industries should embrace geospatial modeling to enhance their decision-making processes. Continuous learning and adaptation to technological advancements will be vital to remain competitive in a rapidly evolving landscape.
FAQ Section
1. What is geospatial modeling?
Geospatial modeling is the process of using spatial data to analyze and represent geographic phenomena, enabling informed decision-making across various sectors.
2. What software is commonly used for geospatial modeling?
Popular software solutions for geospatial modeling include ArcGIS, QGIS, and GRASS GIS, each offering distinct features for data analysis and visualization.
3. How can geospatial modeling help in urban planning?
By analyzing spatial data, geospatial modeling aids urban planners in understanding growth patterns, optimizing land use, and developing sustainable policies for cities.
4. What are spatial data types?
Spatial data types typically include vector data (points, lines, polygons) and raster data (grid cells), each serving different analytical purposes.
5. What are some key applications of geospatial modeling?
Geospatial modeling has applications in urban planning, environmental management, disaster response, transportation planning, and public health.
6. What challenges are associated with geospatial modeling?
Data quality issues, technical complexities, and ethical considerations, such as privacy and data bias, are some of the main challenges in geospatial modeling.
7. How is AI impacting geospatial modeling?
AI enhances geospatial modeling by automating data analysis processes, improving predictive modeling, and assisting in the interpretation of complex datasets.
8. Why is data quality important in geospatial modeling?
High-quality data ensures the accuracy and reliability of modeling outcomes, allowing for better decision-making and resource allocation.
9. What are the benefits of incorporating geospatial modeling into decision-making?
Benefits include enhanced decision-making capabilities, improved management of resources, and the ability to conduct predictive analytics for future planning.
10. How will the future of geospatial modeling look?
Expect ongoing advancements in analytics and visualization tools, increasing integration with IoT, and a strong focus on sustainability and smart city initiatives.
Section | Details |
---|---|
Historical Background | Development of geospatial technologies and tools over time. |
Key Concepts | Understanding spatial data, GIS, and analysis techniques. |
Importance | Applications and benefits across diverse sectors. |
Tools | Overview of popular software and emerging technologies. |
Challenges | Data quality, technical complexities, and ethical implications. |
Future Trends | Innovations and industry outlook for geospatial modeling. |